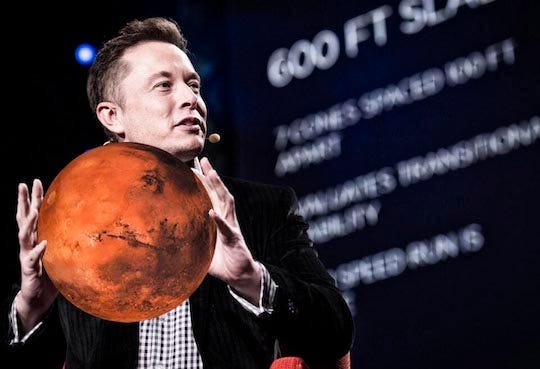
BLOGPOST \ The Idealism of Research on Large Language Models
Owing to their impressively fast development and the enormous cultural impact of Open AI’s ChatGPT, large language models (LLMs) have become a hot topic in the academic landscape. While much interesting work is emerging from that enthusiasm, I also regularly come across a persistent blind spot in these works, something that I would call an unspoken form of idealism, in the Marxist sense. That is, many interventions are implicitly underwritten by a seeming conviction that the world is created through the categories we impose upon it, that social change happens in thought. Only through that hypothesis can I come to make sense of the degree of fascination that I witness for what we could call the ‘culture-production potential’ of LLMs; a fascination that comes at the expenses of other important foci that I associate to a materialist perspective.
This critique could be made of much of the field that endorsed the name of artificial intelligence but the case of LLMs is particularly striking. Indeed, LLMs output natural language that is oftentimes undistinguishable from text produced by a human. As such, the idealist tendency to see culture as the primary site of social change finds a natural focus on that technology: if the machine can ‘speak’, its role in the fabric of culture and thus of the social world, might be overwhelming. Therefore, there are high stakes to improve our understanding of these models.
The interest for the culture-production potential of LLMs shows in the philosophical research about the technology itself. For instances, researchers investigate whether LLMs can be said to have beliefs (Levinstein and Herrmann, 2023); whether the texts they produce can be said to refer to things in the world (Mandelkern and Linzen, 2023), to be grounded, or again to have a symbolic structure (Pavlick, 2023). All these questions share to have at stake the cognitive and/or discursive status of LLMs or their outputs[1]. The importance of such research for idealists is straightforward: if LLMs have things such as beliefs or communicative intents, if their words mean something or refer to the world, this suggests that they can engage in the primary political activity, and thus should be a primary object of political attention.
The idealist inclination of the debates about LLMs becomes particularly clear when we look at the political commentaries that have been made. From The Economist (2022) to a Jacobin article explaining that “ChatGPT Is an Ideology Machine” (2023), commentators across the political board have dedicated their attention to warning us against the great transformations that would come from within the text-generation system. In that regard, Bender et al. (2021) scathing and widely-cited article on the “dangers” of LLMs is particularly telling: virtually all the problems and solutions that they identify boil down to minding the language that goes in and out the models. Most of their attention is on the risks that LLMs might spread language that is “perpetuating dominant viewpoints, increasing power imbalances, and further reifying inequality” (p.614), and even their brief discussion of the environmental impact of LLMs concludes on the question of whether it is “fair” that the Maldives or Sudan should bear the costs of environmental degradation “when [LLMs] aren’t being produced for Dhivehi or Sudanese Arabic?” (pp.612-3). Finally, all the paths forward they propose consist in building the models differently by paying heeds to their culture-production potential.
The issues that Bender et al. (2021) identify strike me as real and relevant, but it also seems to me that the authors are incapable of telling us why these issues arise, and thus what to do about them. For example, it is at best unhelpful to discuss the environmental impact of LLMs without mentioning that their development and use is currently driven by the imperatives of a ruthless commercial competition—global corporate investment in AI has gone up thirteenfold since 2013 (Maslej et al., 2023, p.184)—and that no hot take on linguistic injustice is going to change that. Similarly, it feels off to mention economic inequalities and the condition of marginalised communities without looking at how new technologies impact people’s capacities to reproduce the conditions of their life—for instance, how the training of AI is becoming a substantial source of income in certain countries of the Global South, with labour arrangements that are often highly criticisable (Viana Braz, Tubaro and Casilli, 2023). In short, my view is that we need to remember that LLMs are not just objects or actors of cultural production and put far more emphasis on the social relations that they come to mediate—this is my most general understanding of materialism—least we want to invisibilise the concrete effects that this technology has on people’s lives.
References
Bender, E. M., Gebru, T., McMillan-Major, A., & Shmitchell, S. (2021). On the dangers of stochastic parrots: Can language models be too big? In Proceedings of the 2021 ACM conference on fairness, accountability, and transparency (pp. 610–623).
Jha, A. (2023). How the tech behind ChatGPT could change the world—an updated episode from our archive. The Economist. Retrieved from https://www.economist.com/podcasts/2022/12/27/how-the-tech-behind-chatgpt-could-change-the-world-an-updated-episode-from-our-archive
Levinstein, B., & Herrmann, D. A. (2023). Still no lie detector for language models: Probing empirical and conceptual roadblocks. arXiv preprint.
Mandelkern, M., & Linzen, T. (2023). Do language models refer? arXiv preprint.
Maslej, N., Fattorini, L., Brynjolfsson, E., Etchemendy, J., Ligett, K., Lyons, T., . . . Perrault, R. (2023). The AI index 2023 annual report. In AI Index steering committee, institute for human-centered AI. Standford University.
Pavlick, E. (2023). Symbols and grounding in large language models. Philosophical Transactions of the Royal Society A, 381(2251),
Viana Braz, M., Tubaro, P., & Casilli, A. (2023). Microwork in Brazil: who are the workers behind Artificial Intelligence? In Research report DiPLab & LATRAPS. Retrieved from https://diplab.eu/?p=2833
Weatherby, L. (2023). ChatGPT is an ideology machine. Jacobin. Retrieved from https://jacobin.com/2023/04/chatgpt-ai-language-models -ideology-media-production
[1] Although I don’t mean to claim that these are their only stake.